What Makes Modeling Climate So Difficult?
Climate modeling is an essential tool for understanding and predicting future climate changes. However, the process is fraught with complexities and challenges that make it difficult to develop accurate models. These challenges range from the intricate interactions within the climate system to limitations in data and computational resources, all of which contribute to uncertainties in climate projections.
Complexity of the Climate System
Interactions Between Components
The climate system comprises various interdependent components, including the atmosphere, oceans, land, and biosphere. Each of these elements interacts in complex ways, influencing one another and contributing to the overall climate dynamics. For instance, changes in ocean temperatures can affect atmospheric circulation patterns, which in turn can impact weather systems globally.
Feedback Loops
Feedback mechanisms play a crucial role in climate dynamics. Positive feedbacks, such as the ice-albedo effect (where melting ice reduces reflectivity, leading to further warming), can amplify climate changes. Conversely, negative feedbacks can dampen these changes. The presence of such feedback loops introduces significant uncertainty into predictions, as their effects can be difficult to quantify accurately.
Non-Linear Behavior
The climate system exhibits non-linear behavior, meaning that small changes in one component can lead to disproportionately large effects elsewhere. For example, a slight increase in global temperature can trigger significant shifts in weather patterns or ocean currents, complicating predictive models.
Scale and Resolution Challenges
Global vs. Regional Modeling
While global climate models (GCMs) provide a broad overview of climate trends, they often struggle with regional predictions due to scale limitations. When downscaling these models for local forecasts, critical details may be lost, leading to inaccuracies.
Spatial and Temporal Resolution
Achieving high spatial and temporal resolution in climate models requires immense computational power and extensive data. For example, predicting climate impacts for specific cities necessitates fine-grained models that are currently beyond the capabilities of many existing systems.
Timescale Complexity
Climate modeling typically spans decades to centuries, necessitating long-term data collection and projections based on various emission scenarios. This long-term perspective introduces additional uncertainties regarding future human behavior and technological advancements.
Limitations in Data and Historical Records
Inadequate Historical Data
Historical climate data is often limited or sparse, particularly from earlier centuries or remote regions like the polar areas and deep oceans. This scarcity complicates efforts to establish reliable historical baselines for model validation.
Estimating Prehistoric Climate Conditions
Scientists often rely on indirect data sources—such as ice cores and sediment layers—to infer past climates. These methods introduce uncertainties regarding how accurately past conditions are represented in models.
Data Gaps in Certain Regions
Real-time data collection is particularly challenging in critical regions such as polar areas or deep oceans. The lack of measurements in these areas hinders our understanding of essential climate processes occurring there.
Uncertainty in Future Emissions and Human Behavior
Predicting Human Behavior
One of the most significant challenges in climate modeling is predicting future human behavior regarding emissions. Uncertainties surrounding policy changes, economic growth, and technological advancements complicate projections significantly.
Scenario-Based Modeling
To address these uncertainties, models often utilize various scenarios—such as high or low emissions pathways—to explore potential futures. This approach results in a range of possible outcomes rather than definitive predictions.
Influence of Policy and Innovation
Future policies aimed at reducing emissions or breakthroughs in renewable energy technologies can significantly alter emissions trajectories. This variability adds another layer of complexity to climate modeling efforts.
Computational Limits and Advancing Technology
High Computational Demands
The intricate equations governing climate systems require substantial computational resources for simulations. High-resolution models demand supercomputers capable of processing vast amounts of data simultaneously.
Balancing Accuracy with Efficiency
Modelers often simplify complex processes (like cloud formation) to make calculations feasible within available computational limits. However, this simplification can reduce model accuracy.
Advancements in Machine Learning
Recent advancements in machine learning offer promising avenues for improving climate modeling accuracy. AI techniques are being integrated into existing models to enhance predictive capabilities while addressing issues related to data availability and model interpretability.
Representing Uncertain Factors Like Clouds and Aerosols
Cloud Formation and Behavior
Clouds significantly influence climate by both reflecting sunlight and trapping heat; however, their behavior remains one of the largest uncertainties in modeling efforts due to their complex dynamics.
Aerosol Impact
Aerosols—tiny particles suspended in the atmosphere—can alter temperature by reflecting sunlight or changing cloud properties. Their effects add complexity to short-term climate predictions.
Water Vapor and Greenhouse Effects
Water vapor acts as a potent greenhouse gas that amplifies warming effects; its variability further complicates model predictions due to its dynamic nature within the atmosphere.
The Role of Climate Models Despite Their Challenges
Understanding Climate Trends
Despite inherent uncertainties, climate models provide valuable insights into long-term trends that inform policy decisions and adaptation strategies for mitigating climate impacts.
Refinement Through Model Intercomparison Projects
Projects like the Coupled Model Intercomparison Project (CMIP) help refine models by comparing outputs across different systems, leading to improved accuracy over time.
Role in Assessing Climate Risks
Models are crucial for projecting extreme weather events, sea-level rise, and regional impacts—information that is vital for effective risk management strategies.
Conclusion
Modeling the climate presents numerous challenges stemming from system complexity, data limitations, computational demands, and uncertainties about future human behavior. Despite these difficulties, climate models remain invaluable tools for understanding trends and preparing for future changes. Continued advancements in technology and methodologies will be essential for improving their accuracy and reliability moving forward.
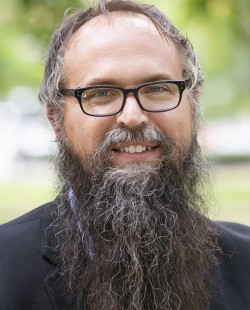
Kyle Whyte is a notable scholar and professor at the University of Michigan, holding positions such as the George Willis Pack Professor in the School for Environment and Sustainability and Professor of Philosophy. Specializing in environmental justice, his work critically examines climate policy and Indigenous peoples’ ethics, emphasizing the nexus between cooperative scientific endeavors and Indigenous justice. As an enrolled Citizen Potawatomi Nation member, he brings a vital perspective to his roles as a U.S. Science Envoy and member of the White House Environmental Justice Advisory Council. His influential research is supported by various prestigious organizations including the National Science Foundation, and disseminated through publications in high-impact journals. Kyle actively contributes to global Indigenous research methodologies and education, with affiliations to numerous institutes and societies dedicated to traditional knowledge and sustainability. Recognized for his academic and community engagement, Kyle has earned multiple awards and served in various visiting professorships. His efforts extend to leadership positions on boards and committees focused on environmental justice nationwide.